Deep Learning and Implementations in Banking
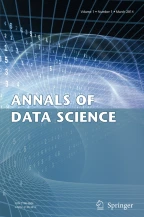
Data-driven technologies have been changing every aspect of human life and the fast-developing banking sector with its data-rich nature has become the implementation field of these fast-evolving technologies. Deep learning, as one of the emerging technologies in recent years, has also been inevitably adopted for various improvements in banking. To the best of our knowledge, there is no comprehensive literature review, which focuses on specifically deep learning and its implementations in banking. Therefore, this paper investigates the deep learning technology in-depth and summarizes the relevant applications in banking so to contribute to the existing literature. Moreover, by providing a reliable and up-to-date review, it is also aimed to serve as the one-stop repository for banks and researchers who are interested in embracing deep learning, whilst bringing insights for the directions of future research and implementation.
This is a preview of subscription content, log in via an institution to check access.
Access this article
Subscribe and save
Springer+ Basic
€32.70 /Month
- Get 10 units per month
- Download Article/Chapter or eBook
- 1 Unit = 1 Article or 1 Chapter
- Cancel anytime
Buy Now
Price includes VAT (France)
Instant access to the full article PDF.
Rent this article via DeepDyve
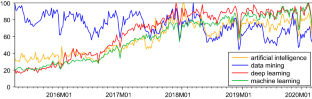
Similar content being viewed by others
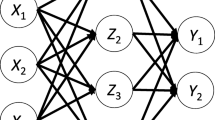
Deep learning in finance and banking: A literature review and classification
Article Open access 08 June 2020
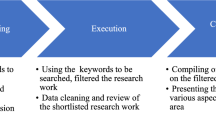
Is artificial intelligence and machine learning changing the ways of banking: a systematic literature review and meta analysis
Article Open access 04 December 2023
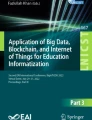
A Survey of Trendy Financial Sector Applications of Machine and Deep Learning
Chapter © 2023
Explore related subjects
References
- Saini A, Sharma A (2019) Predicting the unpredictable: an application of machine learning algorithms in indian stock market. Ann Data Sci. https://doi.org/10.1007/s40745-019-00230-7ArticleGoogle Scholar
- Ahmed M, Najmul Islam AKM (2020) Deep learning: hope or hype. Ann Data Sci. https://doi.org/10.1007/s40745-019-00237-0ArticleGoogle Scholar
- Xu Z, Shi Y (2015) Exploring big data analysis: fundamental scientific problems. Ann Data Sci 2:363–372 Google Scholar
- Shi Y, Shan Z, Li J, Jianping L, Fang Y (2017) How China deals with big data. Ann Data Sci 4:433–440 Google Scholar
- Hassani H, Huang X, Silva ES, Ghodsi M (2016) A review of data mining applications in crime. Stat Anal Data Min ASA Data Sci J 9(3):139–154 Google Scholar
- Hassani H, Huang X, Ghodsi M (2018) Big data and causality. Ann Data Sci 5(2):133–156 Google Scholar
- Olson D, Shi Y (2007) Introduction to business data mining. McGraw-Hill/Irwin, New York Google Scholar
- Shi Y, Tian YJ, Kou G, Peng Y, Li JP (2011) Optimization based data mining: theory and applications. Springer, London Google Scholar
- Shi Y (2014) Big Data: history, current status, and challenges going forward. Bridge US Natl Acad Eng 44(4):6–11 Google Scholar
- Hassani H, Silva E (2018) Big Data: a big opportunity for the petroleum and petrochemical industry. OPEC Energy Rev 42(1):74–89 Google Scholar
- Hassani H, Huang X, Silva E (2019) Big Data and climate change. Big Data Cognit Comput 3(1):12 Google Scholar
- Hassani H, Silva ES, Unger S, TajMazinani M, Mac Feely S (2020) Artificial Intelligence (AI) or Intelligence Augmentation (IA): what Is the Future? AI 1:143–155 Google Scholar
- Hassani H, Huang X, Silva E (2018) Digitalisation and big data mining in banking. Big Data Cognit Comput 2(3):18 Google Scholar
- Hassani H, Huang X, Silva E (2018) Banking with blockchain-ed big data. J Manag Anal 5(4):256–275 Google Scholar
- Hormozi AM, Giles S (2004) Data mining: a competitive weapon for banking and retail industries. Inf Syst Manag 21(2):62–71 Google Scholar
- Chitra K, Subashini B (2013) Data mining techniques and its applications in banking sector. Int J Emerg Technol Adv Eng 3(8):219–226 Google Scholar
- Jayasree V, Balan RVS (2013) A review on data mining in banking sector. Am J Appl Sci 10(10):1160 Google Scholar
- Chye KH, Gerry CKL (2002) Data mining and customer relationship marketing in the banking industry. Singap Manag Rev 24(2):1–28 Google Scholar
- Aburrous M, Hossain MA, Dahal K, Thabtah F (2010) Intelligent phishing detection system for e-banking using fuzzy data mining. Expert Syst Appl 37(12):7913–7921 Google Scholar
- Ince H, Aktan B (2009) A comparison of data mining techniques for credit scoring in banking: a managerial perspective. J Bus Econ Manag 10(3):233–240 Google Scholar
- Sun N, Morris JG, Xu J, Zhu X, Xie M (2014) iCARE: a framework for big data-based banking customer analytics. IBM J Res Dev 58(5/6):4:1–4:9 Google Scholar
- Srivastava U, Gopalkrishnan S (2015) Impact of big data analytics on banking sector: learning for Indian banks. Procedia Comput Sci 50:643–652 Google Scholar
- Tsai CF, Chen ML (2010) Credit rating by hybrid machine learning techniques. Appl Soft Comput 10(2):374–380 Google Scholar
- Khandani AE, Kim AJ, Lo AW (2010) Consumer credit-risk models via machine-learning algorithms. J Bank Finance 34(11):2767–2787 Google Scholar
- Carr M, Ravi V, Reddy GS, Veranna D (2013) Machine learning techniques applied to profile mobile banking users in India. Int J Inf Syst Serv Sect 5(1):82–92 Google Scholar
- Smeureanu I, Ruxanda G, Badea LM (2013) Customer segmentation in private banking sector using machine learning techniques. J Bus Econ Manag 14(5):923–939 Google Scholar
- Hassani H, Huang X, Silva ES (2019) Fusing Big Data, blockchain, and cryptocurrency. In: Fusing Big Data, blockchain and cryptocurrency, Palgrave Pivot
- Domingos P (2012) A few useful things to know about machine learning. Commun ACM 55(10):78–87 Google Scholar
- Grossfeld B (2020) Deep learning vs machine learning: a simple way to understand the difference. Available online: https://www.zendesk.com/blog/machine-learning-and-deep-learning/, Accessed 20 Mar 2020
- Alpaydin E (2020) Introduction to machine learning. MIT Press, Cambridge Google Scholar
- Shalev-Shwartz S, Ben-David S (2014) Understanding machine learning: from theory to algorithms. Cambridge University Press, Cambridge Google Scholar
- LeCun Y, Bengio Y, Hinton G (2015) Deep learning. Nature 521(7553):436–444 Google Scholar
- Guo Y, Liu Y, Oerlemans A, Lao S, Wu S, Lew MS (2016) Deep learning for visual understanding: a review. Neurocomputing 187:27–48 Google Scholar
- Zhu XX, Tuia D, Mou L, Xia GS, Zhang L, Xu F, Fraundorfer F (2017) Deep learning in remote sensing: a comprehensive review and list of resources. IEEE Geosci Remote Sens Mag 5(4):8–36 Google Scholar
- Miotto R, Wang F, Wang S, Jiang X, Dudley JT (2018) Deep learning for healthcare: review, opportunities and challenges. Brief Bioinf 19(6):1236–1246 Google Scholar
- Fawaz HI, Forestier G, Weber J, Idoumghar L, Muller PA (2019) Deep learning for time series classification: a review. Data Min Knowl Discov 33(4):917–963 Google Scholar
- Fan C, Xiao F, Zhao Y (2017) A short-term building cooling load prediction method using deep learning algorithms. Appl Energy 195:222–233 Google Scholar
- Socher R, Lin CC, Manning C, Ng AY (2011) Parsing natural scenes and natural language with recursive neural networks. In: Proceedings of the 28th international conference on machine learning (ICML-11), pp 129–136
- Mikolov T, Karafiát M, Burget L, Černockỳ J, Khudanpur S (2010) Recurrent neural network based language model. In: Eleventh annual conference of the international speech communication association
- Hochreiter S, Schmidhuber J (1997) Long short-term memory. Neural Comput 9(8):1735–1780 Google Scholar
- Szegedy C, Liu W, Jia Y, Sermanet P, Reed S, Anguelov D, Erhan D, Vanhoucke V, Rabinovich A (2015) Going deeper with convolutions. In Proceedings of the IEEE conference on computer vision and pattern recognition, pp. 1–9
- Simonyan K, Zisserman A (2014) Very deep convolutional networks for large-scale image recognition. arXiv preprint arXiv:1409.1556
- Chollet F (2017) Xception: Deep learning with depthwise separable convolutions. In Proceedings of the IEEE conference on computer vision and pattern recognition, pp 1251–1258
- LeCun Y, Bottou L, Bengio Y, Haffner P (1998) Gradient-based learning applied to document recognition. Proc IEEE 86(11):2278–2324 Google Scholar
- Donahue J, Jia Y, Vinyals O, Hoffman J, Zhang N, Tzeng E, Darrell T (2014) Decaf: a deep convolutional activation feature for generic visual recognition. In: International conference on machine learning, pp 647–655
- Salakhutdinov R, Hinton G (2009) Deep boltzmann machines. In: Artificial intelligence and statistics, pp 448–455
- Schmidhuber J (2015) Deep learning in neural networks: an overview. Neural Netw 61:85–117 Google Scholar
- Pouyanfar S, Sadiq S, Yan Y, Tian H, Tao Y, Reyes MP, Shyu ML, Chen SC, Iyengar SS (2018) A survey on deep learning: algorithms, techniques, and applications. ACM Comput Surv (CSUR) 51(5):1–36 Google Scholar
- Liu W, Wang Z, Liu X, Zeng N, Liu Y, Alsaadi FE (2017) A survey of deep neural network architectures and their applications. Neurocomputing 234:11–26 Google Scholar
- Bengio Y, Lamblin P, Popovici D, Larochelle H (2007) Greedy layer-wise training of deep networks. In: Advances in neural information processing systems, pp 153–160
- Vincent P, Larochelle H, Bengio Y, Manzagol PA (2008) Extracting and composing robust features with denoising autoencoders. In: Proceedings of the 25th international conference on Machine learning, pp 1096–1103
- Kingma DP, Welling M (2013) Auto-encoding variational bayes. arXiv preprint arXiv:1312.6114
- Goodfellow I, Pouget-Abadie J, Mirza M, Xu B, Warde-Farley D, Ozair S, Courville A, Bengio Y (2014) Generative adversarial nets. In: Advances in neural information processing systems, pp 2672–2680
- Bose I, Chen X (2009) Quantitative models for direct marketing: a review from systems perspective. Eur J Oper Res 195(1):1–16 Google Scholar
- Sing’oei L, Wang J (2013) Data mining framework for direct marketing: a case study of bank marketing. Int J Comput Sci Issues 10((2 Part 2)):198 Google Scholar
- Kim KH, Lee CS, Jo SM, Cho SB (2015) Predicting the success of bank telemarketing using deep convolutional neural network. In: 2015 7th international conference of soft computing and pattern recognition (SoCPaR), IEEE, pp 314–317
- Zakaryazad A, Duman E (2016) A profit-driven Artificial Neural Network (ANN) with applications to fraud detection and direct marketing. Neurocomputing 175:121–131 Google Scholar
- Moro S, Cortez P, Rita P (2014) A data-driven approach to predict the success of bank telemarketing. Decis Support Syst 62:22–31 Google Scholar
- Yan C (2018) Convolutional Neural Network on a structured bank customer data. Towards data science. Available online: https://towardsdatascience.com/convolutional-neural-network-on-a-structured-bank-customer-data-358e6b8aa759, Accessed on 25 Mar 2020
- Ładyżyński P, Żbikowski K, Gawrysiak P (2019) Direct marketing campaigns in retail banking with the use of deep learning and random forests. Expert Syst Appl 134:28–35 Google Scholar
- Ogwueleka FN, Misra S, Colomo-Palacios R, Fernandez L (2015) Neural network and classification approach in identifying customer behavior in the banking sector: a case study of an international bank. Hum Factors Ergon Manuf Serv Ind 25(1):28–42 Google Scholar
- Davies F, Moutinho L, Curry B (1996) ATM user attitudes: a neural network analysis. Mark Intell Plan 14:26–32 Google Scholar
- Hsieh NC (2004) An integrated data mining and behavioral scoring model for analyzing bank customers. Expert Syst Appl 27(4):623–633 Google Scholar
- Laukkanen T, Pasanen M (2008) Mobile banking innovators and early adopters: How they differ from other online users? J Financ Serv Mark 13(2):86–94 Google Scholar
- Wang G, Bai Y, Sun Y (2018) Application of BP neural network algorithm in bank customer hierarchy system. In: 2017 3rd international forum on energy, environment science and materials (IFEESM 2017), Atlantis Press
- Zhou X, Bargshady G, Abdar M, Tao X, Gururajan R, Chan KC (2019) A case study of predicting banking customers behaviour by using data mining. In: 2019 6th international conference on behavioral, economic and socio-cultural computing (BESC), IEEE, pp 1–6
- Vieira A, Sehgal A (2018) How banks can better serve their customers through artificial techniques. In: Digital marketplaces unleashed, Springer, Berlin, pp 311–326
- Krishnan K (2020) Chapter 7—Banking industry applications and usage. Building Big Data Applications, Academic Press, pp 127–144
- Marous J (2018) Meet 11 of the most interesting chatbots in banking. The Financial Brand. Available online: https://thefinancialbrand.com/71251/chatbots-banking-trends-ai-cx/, Accessed on 26 Mar 2020
- Quah JT, Chua YW (2019) Chatbot Assisted Marketing in Financial Service Industry. In: International conference on services computing, Springer, pp 107–114
- Przegalinska A, Ciechanowski L, Stroz A, Gloor P, Mazurek G (2019) In bot we trust: a new methodology of chatbot performance measures. Bus Horiz 62(6):785–797 Google Scholar
- Spanoudes P, Nguyen T (2017) Deep learning in customer churn prediction: unsupervised feature learning on abstract company independent feature vectors. arXiv preprint arXiv:1703.03869
- Mirashk H, Albadvi A, Kargari M, Javide M, Eshghi A, Shahidi G (2019) Using RNN to predict customer behavior in high volume transactional data. In: International congress on high-performance computing and big data analysis, Springer, pp 394–405
- De Caigny A, Coussement K, De Bock KW, Lessmann S (2019) Incorporating textual information in customer churn prediction models based on a convolutional neural network. Int J Forecast (In Press)
- Leo M, Sharma S, Maddulety K (2019) Machine learning in banking risk management: a literature review. Risks 7(1):29 Google Scholar
- Petropoulos A, Siakoulis V, Stavroulakis E, Vlachogiannakis NE (2020) Predicting bank insolvencies using machine learning techniques. Int J Forecast. https://doi.org/10.1016/j.ijforecast.2019.11.005 In Press ArticleGoogle Scholar
- Lin WY, Hu YH, Tsai CF (2011) Machine learning in financial crisis prediction: a survey. IEEE Trans Syst Man Cybern Part C Appl Rev 42(4):421–436 Google Scholar
- Sezer OB, Gudelek MU, Ozbayoglu AM (2020) Financial time series forecasting with deep learning: a systematic literature review: 2005–2019. Appl Soft Comput 90:106181 Google Scholar
- Karisma H, Widyantoro DH (2016) Comparison study of neural network and deep neural network on repricing GAP prediction in Indonesian conventional public bank. In: 2016 6th international conference on system engineering and technology (ICSET), IEEE, pp 116–122
- Culkin R, Das SR (2017) Machine learning in finance: the case of deep learning for option pricing. J Invest Manag 15(4):92–100 Google Scholar
- Weigand A (2019) Machine learning in empirical asset pricing. Financ Mark Portf Manag 33(1):93–104 Google Scholar
- Chen Y, Rabbani RM, Gupta A, Zaki MJ (2017) Comparative text analytics via topic modeling in banking. In: 2017 IEEE symposium series on computational intelligence (SSCI), IEEE, pp 1–8
- Rönnqvist S, Sarlin P (2017) Bank distress in the news: describing events through deep learning. Neurocomputing 264:57–70 Google Scholar
- Mai F, Tian S, Lee C, Ma L (2019) Deep learning models for bankruptcy prediction using textual disclosures. Eur J Oper Res 274(2):743–758 Google Scholar
- Qu Y, Quan P, Lei M, Shi Y (2019) Review of bankruptcy prediction using machine learning and deep learning techniques. Procedia Comput Sci 162:895–899 Google Scholar
- Vo NN, He X, Liu S, Xu G (2019) Deep learning for decision making and the optimization of socially responsible investments and portfolio. Decis Support Syst 124:113097 Google Scholar
- Luo C, Wu D, Wu D (2017) A deep learning approach for credit scoring using credit default swaps. Eng Appl Artif Intell 65:465–470 Google Scholar
- Sirignano J, Sadhwani A, Giesecke K (2018) Deep learning for mortgage risk. Available at: https://doi.org/10.2139/ssrn.2799443
- Addo PM, Guegan D, Hassani B (2018) Credit risk analysis using machine and deep learning models. Risks 6(2):38 Google Scholar
- Kvamme H, Sellereite N, Aas K, Sjursen S (2018) Predicting mortgage default using convolutional neural networks. Expert Syst Appl 102:207–217 Google Scholar
- Gomez JA, Arevalo J, Paredes R, Nin J (2018) End-to-end neural network architecture for fraud scoring in card payments. Pattern Recognit Lett 105:175–181 Google Scholar
- Fu K, Cheng D, Tu Y, Zhang L (2016) Credit card fraud detection using convolutional neural networks. In: International conference on neural information processing, Springer, pp 483–490
- Zhang Z, Zhou X, Zhang X, Wang L, Wang P (2018) A model based on convolutional neural network for online transaction fraud detection. Secur Commun Netw 2:1–9 Google Scholar
- Kazemi Z, Zarrabi H (2017) Using deep networks for fraud detection in the credit card transactions. In: 2017 IEEE 4th international conference on knowledge-based engineering and innovation (KBEI), IEEE, pp 0630–0633
- Zamini M, Montazer G (2018) Credit card fraud detection using autoencoder based clustering. In: 2018 9th international symposium on telecommunications (IST), IEEE, pp 486–491
- Pumsirirat A, Yan L (2018) Credit card fraud detection using deep learning based on auto-encoder and restricted boltzmann machine. Int J Adv Comput Sci Appl 9(1):18–25 Google Scholar
Author information
Authors and Affiliations
- Research Institute for Energy Management and Planning, University of Tehran, Tehran, Iran Hossein Hassani & Mansi Ghodsi
- Department of Strategic Management and Marketing, De Montfort University, Leicester, UK Xu Huang
- London College of Fashion, University of the Arts London, London, UK Emmanuel Silva
- Hossein Hassani